Statistics for Machine Learning

Enroll in this statistics for machine learning course and its correlation analysis. Get ready for this interesting sesison by Dr. Abhinanda Sarkar, and give your career a success in the ML domain.
What you learn in Statistics for Machine Learning ?






About this Free Certificate Course
An understanding of basic statistics for machine learning concepts provides a strong foundation for further learning in the fields of data analysis, data science, and even some areas of machine learning. Without statistics, it becomes nearly impossible to work on becoming an expert in this domain when working with real-time or industry-grade products. Hence, to understand the domain and actively implement it, statistics is very much the need of the hour for Machine Learning.
This free online statistics in machine learning course covers the basics of descriptive statistics and data visualizations. You will be learning about the importance of this functional concept called statistics in this vast domain. Statistics is a key requirement which acts as a foundation to build up for further concepts down the line, hence it makes it very vital that you understand this. It also explains the various kinds of statistical distributions and how to apply them to business problems in a simple manner.
The University of Texas at Austin, in collaboration with Great Lakes Executive Learning, offers several Post Graduate courses in the field of Artificial Intelligence. Explore more about our Artificial Intelligence Course and enroll in it to earn a Postgraduate Certificate in the Artificial Intelligence and Machine Learning online course from the University of Texas and Great Lakes Executive Learning. This course is #1 ranked in India, which ensures you become a successful AI/ML professional with a comprehensive curriculum and industry-relevant projects.
Check out our PG Course in Machine learning Today.
Course Outline
Our course instructor
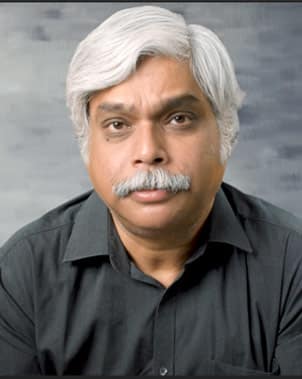
Dr. Abhinanda Sarkar
Faculty Director, Great Learning


Dr. Sarkar’s publications, patents, and technical leadership have been in applying probabilistic models, statistical data analysis, and machine learning to diverse areas such as experimental physics, computer vision, text mining, wireless networks, e-commerce, credit risk, retail finance, engineering reliability, renewable energy, and infectious diseases, His teaching has mostly been on statistical theory, methods, and algorithms; together with application topics such as financial modeling, quality management, and data mining.
Dr. Sarkar is a certified Master Black Belt in Lean Six Sigma and Design for Six Sigma. He has been visiting faculty at Stanford and ISI and continues to teach at the Indian Institute of Management (IIM-Bangalore) and the Indian Institute of Science (IISc). Over the years, he has designed and conducted numerous corporate training sessions for technology and business professionals. He is a recipient of the ISI Alumni Association Medal, IBM Invention Achievement Awards, and the Radhakrishan Mentor Award from GE India
With this course, you get
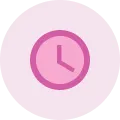
Free lifetime access
Learn anytime, anywhere
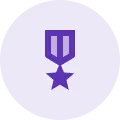
Completion Certificate
Stand out to your professional network
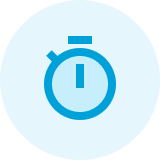
2.0 Hours
of self-paced video lectures
Frequently Asked Questions
Is statistics required for machine learning?
Yes, statistics are very important for machine learning. As mentioned above, statistics is applied to the following machine learning tasks and phases:
- Framing the problem
- Understanding the data
- Data Cleaning
- Data Selection
- Data Preparation
- Model Evaluation
- Model Configuration
- Model Selection
- Model Presentation
- Model Predictions
What are some prerequisites to learn Statistics for Machine Learning?
To learn statistics for machine learning, you might have to need a certain skill set, including:
- Basic statistics, calculus, linear algebra, and probability. The statistics include all the tools used to achieve an outcome from the input data.
- Proficient programming knowledge. Being able to write code is an essential skill since it is a fundamental skill in machine learning.
- Good knowledge in data modeling
What statistics should I know for machine learning?
The basics of statistics are extremely important for working with machine learning models and algorithms. Other statistical methods important for machine learning are Hypothesis Testing, Bayes' Theorem, Binomial Distribution, Poisson Distribution, Normal Distribution.
What is statistical learning in machine learning?
The knowledge of statistical methods that are crucial for working on machine learning models and functional analysis is known as statistical learning. The Statistical learning theory deals with the problem of finding a predictive function based on data.
Is machine learning better than statistics?
You cannot draw a comparison between the two domains as machine learning depends on statistical methods for many functions and tasks.
Success stories
Can Great Learning Academy courses help your career? Our learners tell us how.And thousands more such success stories..
Related Machine Learning Courses
Popular Upskilling Programs
Explore new and trending free online courses
Relevant Career Paths >
Statistics for Machine Learning Course
Machine Learning is an interdisciplinary field that includes applications of probability, algorithms, and statistics to make sense of the huge pool of data. The field of study involves identifying insights from data to build intelligent models.
What is Statistics?
Statistics is a specialised field of study in mathematics. It is a collection of different methods that are used to answer specific questions by working with available data. The definition of statistics by the book is, “Statistics is the art of making numerical conjectures about puzzling questions. The methods were developed over several hundred years by people who were looking for answers to their questions.”
Why should you learn Statistics?
The raw data collected from various sources itself does not hold any value until it is processed, studied, and made sense of. Also, raw observations are not knowledge or information. Therefore, statistics is important to draw inferences from the data for improving existing processes and methods and find patterns for forecasting.
Statistics is used to answer the following questions from a pool of data:
- Which is the most expected observation?
- What are the limits to the observations?
- What does the data look like?
- What is the relevance of each variable?
- What are the differences in the outcomes of multiple experiments?
- Are these differences genuine or the results of noise?
Such questions might sometimes look simple or irrelevant, but should be answered to transform raw data into information that could be crucial for business decisions. Also, these questions matter to the project, the teams, and the stakeholders. In short, statistical methods are required to find answers to the questions that we have about data.
Descriptive Statistics
Descriptive statistics include the methods that summarise the raw observations into useful information that is understandable and shareable. It deals with the calculation of statistical values on samples of data to summarise the properties of the sample data. These values or properties include the mean, median, variance, and standard deviation.
The descriptive statistics also cover the graphical methods used for data visualisation. Data visualisation provides a better understanding of the distribution and the relationship between the variables.
Inferential Statistics
Inferential statistics aid in quantifying properties of the population from a smaller sample data set. It is commonly thought to be the estimation of the quantities from the population distribution. These could be expected value or the amount of spread.
More sophisticated statistical inference tools are the statistical hypothesis testing where the base assumption of the test is called the null hypothesis.
How is Machine Learning Used in Statistics
Statistics for Machine Learning is used in the following ways:
- Framing the problem
- Understanding the data
- Data Cleaning
- Data Selection
- Data Preparation
- Model Evaluation
- Model Configuration
- Model Selection
- Model Presentation
- Model Predictions
1. Framing the problem
Problem framing essentially means the selection of the type of problem, i.e. classification or regression. Also, the selection of types of input and output for the problem comes under problem framing.
For freshers in the field of machine learning, problem framing could be a challenging task as it requires a thorough exploration of the observations and data collected. On the other hand, for the experienced folks, they may benefit substantially by considering the data from multiple perspectives using statistical methods.
Exploratory data analysis and Data mining techniques are the commonly used statistical methods in the problem framing stage.
2. Understanding the data
Data understanding essentially means the clarity with distributions, knowledge of variables, and the relationship these variables have among themselves.
The two common statistical methods used in understanding data are summary statistics and data visualisation.
3. Data Cleaning
The data collected through various digital channels are often subjected to processes that can damage its fidelity. Some of the examples that tarnish originality of the data are data corruption, loss of data, and errors in data. Therefore, it is important to clean the data and repair the issues with this data.
The statistical methods that are used for data cleaning purposes are outlier detection and feature selection methods.
4. Data Selection
Some of the variables or data might be irrelevant to the model being worked on. In such cases, the scope of the data is reduced to the elements that are most critical for making accurate predictions. This process is known as data selection.
The statistical methods used for the purpose of data selection are Data Sample and Feature Selection.
5. Data Preparation
Data needs some preparation before being used for modeling. This stage involves changing the shape or structure of the data to make it more suitable for the problem at hand. Scaling, Encoding, and Transforms are some of the statistical methods for machine learning that are used for data preparation.
6. Model Evaluation
Evaluating a learning method is a crucial step in a predictive modeling problem. The planning of the process of training and evaluation of a predictive model is called experimental design which is a sub-fled of statistics.
For implementing an experimental design, resampling methods are used to resample a dataset to make economic use of available data.
Statistics and machin learning go hand in hand. The other areas where statistics is used in machine learning are model configuration, model selection, and model predictions. These are the advanced stages in machine learning about which we will learn later in an advanced level article.
About the Program - Statistics for Machine Learning
The statistics for machine learning course at Great Learning Academy will build a strong foundation for learners who wish to pursue data analysis, data science, and ofcourse machine learning. This free online statistics course curriculum will cover the basics of descriptive statistics, and more advanced concepts such as Baye’s theorem and Hypothesis Testing. It will also cover the various kinds of statistical distributions and how to apply them to real-world problems.
If you wish to learn statistics online, this is the best program for you to start with as it tops the charts among the free online statistics course certificates. The duration of the program is 6.5 hours in the form of video content. At the end, the course also has a quiz for you to measure your learning and claim your certificate.
The detailed course curriculum of the statistics for machine learning course includes Introduction to Statistics, Importance of Statistics, Big Data basics, Data Visualisation, Frequency Distribution and plots, Mean, Median, Mode, Measures of Dispersion, Standard Deviation, Boxplots, Probability Distributions, Baye’s Theorem, Binomial and Poisson Distributions using Python, Normal Distribution in Excel and Python, and Hypothesis Testing.
The testimonials speak volumes about this course, so head to the testimonial section and check out the value this course adds to one’s learning curve and career.